Table of Contents
Chapter 2 - Why fMRI? Neuroimaging and the movement toward multidisciplinary science
Neuroimaging and the `common language’ of the brain
Human neuroimaging, especially fMRI and PET, is a growing new field now with thousands of publications per year. Why all the excitement? One of the goals of neuroimaging is a movement towards multidisciplinary science. This is one thing we’re particularly excited about. For many years, people in different fields have been studying diverse aspects of the mind, the brain, and the body. Psychologists study the mind and behavior while neuroscientists study the brain. Medical and clinical researchers study the treatment and prevention of illness including those of the mind and the brain, which we increasingly understand to be interconnected with other body systems. Clinical trials study health related interventions and biologists study living systems. The fields of statistics, engineering, and computer science have each emerged as leading disciplines in the study of complex computational and biological processes with different traditions of techniques and approaches.
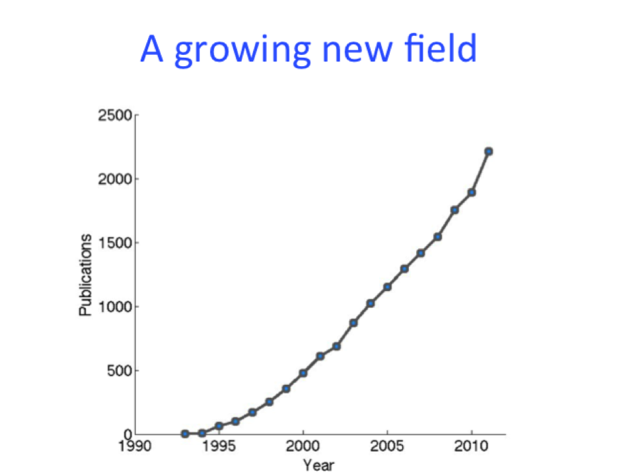
These fields form rich but largely separate traditions. This is in some sense inevitable as a field grows and matures with a strongly shared history of knowledge and increasingly specialized techniques among its practitioners. This canalization and deepening of roots is complemented by new growth of fields that evolve at the intersections among established fields before developing their own research traditions. Psychophysiologists study the mind as related to peripheral physiology. Neuroimmunologists study the brain as related to the immune system. Psychoneuroimmunologists study intersections of the mind, brain, and immune functions. Each of these disciplines provides a crucial but incomplete window into the most exciting frontier in contemporary science: the study of the mind and the brain - the study of us.
There is an old story about a group of blind people who each feel an elephant and try to understand together what they are observing. One person feels something long, rubbery, and flexible. Another perceives a smooth, firm surface and a third identifies a flat, delicate membrane. The study of the mind and the brain is a really, really big elephant. Its study spans several dimensions of analysis. One is a dimension of scale ranging from molecules to cells to systems. Another is a dimension of time from the opening and closing of ion channels in nanoseconds to the long-term relationships between brain and mind over a human lifetime or perhaps over the lifespan of a culture or a species. A third is a dimension of abstractness from concrete physiology to our capacities for abstract thought and emotion: for love, hope, cruelty, and empathy. Each discipline brings something unique to the table, but each specializes in a different ``piece of the elephantее. To understand the whole image, we need to study these pieces deeply and rigorously, and then put them together into a picture of the integrated function of the human brain, mind, body, and environment.
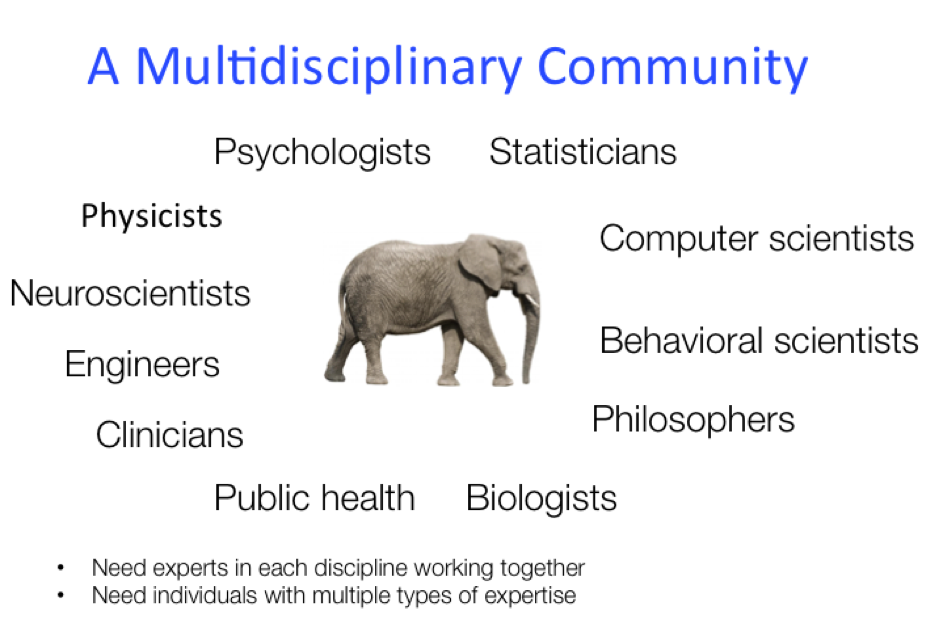
The potential for such integration is one of the most exciting things about fMRI as a technique. Not only does the technology for collecting fMRI data draw on knowledge and techniques from at least a half dozen disciplines but fMRI can also be used to study just about anything related to the brain and the mind. This includes everything from abstract thought to cognitive performance, to mental illness and psychopathology, to brain regulation of inflammation in the body. For a practitioner to integrate the information and techniques required to do these studies well draws on knowledge from dozens of other disciplines.
fMRI and other types of neuroimaging also provide a way for practitioners of different disciplines to come together and speak in the ``common language’’ of the brain. For example, consider a neuroscientist studying the molecular basis of learning, a pharmacologist interested in antipsychotic drugs, a psychiatrist examining depression, and a social psychologist investigating the nature of altruistic behavior. What do all these researchers have in common and what could they possibly converse about relating to each of their core scientific interests? Why, the dopamine system, of course! It is very likely each of these researchers has been studying brain processes related to the mesolimbic dopamine system, which connects the midbrain, ventral striatum, and prefrontal cortex. The researchers each might have results related to brain activity in the ventral striatum that could help inform the others’ ideas about what the system is doing in relation to their outcomes of interest.
Neuroimaging research can even help establish bridges between researchers in the same field who didn’t realize their ideas were grounded in similar neurophysiological processes. For example, some social psychologists study motivation and appetite, others the effects of psychological distance, still others emotion regulation, and another group stereotyping and prejudice. All these areas contain a proliferation of theories, many of which include specific names and concepts (e.g., ``construal level theory’’). How do the mechanisms underlying these theories relate? Do some rely on the same core processes and systems and, if so, what are they and how are they related? Once again, the ventral striatum and medial prefrontal cortex likely play prominent roles in all these areas of social psychological inquiry. Grounding theories in models of brain function can help establish premises in measurable processes. These theories can then be shared across researchers and fields to facilitate building a cumulative science of social cognition and behavior.
Multiple roles, multiple fields: An example
Letеs look at some of the unique roles different disciplines play in an fMRI study by using an example of a basic fMRI study on how antidepressants work. Yes, we still don’t really know much about how antidepressants, opioids, or any of the other systemic drugs (which we have been administering for decades or longer) work. This is in large part because these drugs affect neurons and glia all over the brain and we don’t know much about the effects on the various systems that support thought, emotion, and decision-making. We don’t even have a good consensus on which brain systems sustain those processes and which implement basic functions like attention, learning, and emotion. We do know a lot, but - to continue with our example - if we find that an antidepressant affects the prefrontal cortex, it is difficult to say what that means regarding the course of a personеs mental health or their life.
So, back to our study - we won’t try to solve the whole mystery at once. Rather, this study will simply seek to establish which brain regions change with antidepressant treatment in order to test whether the drugs do indeed alter the function of the prefrontal cortex and other brain regions. The psychologist uses expertise in experimental design to construct a task which can isolate particular mental processes related to depression. The psychologist and statistician both have expertise in ascertaining that the design is efficient and well powered, and that it will produce valid causal inferences about the effects of the drug on the brain. A pharmacologist has information about the cellular and molecular mechanisms of the drug’s action and the kinetics of its absorption into brain tissue; the pharmacologist possibly also has data about its effects on brain vasculature and blood gas levels that may produce artifacts. A psychiatrist knows how drug dose and time course relate to expected clinical efficacy. A neuroscientist may have unique knowledge about how the drug penetrates into the brain and about the effects on neurons, glia, and/or various neural systems. The right training uniquely positions an MR physicist or biomedical engineer to ensure that we can obtain high-quality functional and structural images, and ideally minimize artifacts in the brain areas about which we care the most. The physicist or biomedical engineer may also have crucial information about how vascular and physiological drug effects might impact the fMRI signal independent of neural function. A computer scientist can manage and process the potentially huge volume of data acquired during the experiment, likely by borrowing signal processing techniques from mathematics and electrical engineering. During data analysis, the statistician again plays a critical role in examining the data and the assumptions underlying the statistical tests, ultimately giving us a (hopefully valid!) picture of which brain areas the drug affects. A neuroanatomist can help localize the effects that emerge. The neuroscientist’s purview, together with the psychologist and psychiatrist, is interpreting the results and their meaning.
That provides an overview of the different roles and contributions of various fields in an fMRI study. This description does not imply we need a team of 12 experts to do the study б in fact, that would be highly impractical. For the best science, we need collaboration of experts in multiple disciplines and individuals with proficiency in diverse aspects of design, analysis, and interpretation. A scientist using fMRI might come from any one of these disciplines, but likely has some capability in nearly all of them. While it’s probably impossible to truly be an expert in each of these areas, a good scientist will know something about all of them, have some idea about what she or he doesnеt know, and recognize when and how to ask for advice from colleagues.
A confluence is the running together of rivers into a greater river. This is what the collaboration of disciplines is like: many great rivers running together with their ideas and techniques intermingling and combining. This process is very good for both science and society far beyond the immediate applications of fMRI. This confluence can help those who learn and practice collaboration become educated in a rich set of scientifically grounded ideas. It can lead to new ways of thinking about the mind, health, and disease.
Challenges and motivation for multidisciplinary science
All this sounds great, right? The catch is that it’s actually not easy for people from different disciplines to work together because they must learn and talk about unfamiliar concepts and be willing to not be the expert. Collaboration requires scientists from different disciplines to care about ideas and problems outside the scope of their defined interests and perhaps to publish in journals unfamiliar to or not prestigious in their particular field (very few journals are prestigious across all fields). It also requires time spent educating other team members about basic concepts which are not groundbreaking within one’s own discipline but which may be crucial and perhaps innovative in the context of interdisciplinary science.
For example, many MRI physicists are rewarded for innovating new methods to acquire data, not for explaining the basics of tried-and-true clinical study methods like our example above or for spending time tweaking those methods to minimize the artifacts in the brain structures which impact neuroscientists. Those who are willing to talk to the rest of us should be treated like gold, as should statisticians and others with specialized knowledge to contribute.
So how do we get people to talk to one another and work together? One answer lies in individual scientists developing multiple types of expertise, so that the gulf between the psychologist and the physicist, or the pharmacologist and the statistician, is not so great that they have nothing to say to one another. ``Bridge’’ scientists are the glue that holds the team together. A little knowledge goes a long way in that respect, just like knowing a few words of someone else’s language can produce a dramatically different social interaction than sharing no words. Offering a route to develop expertise is one of the reasons we wanted to write this book.
Another answer is the movement towards multidisciplinary science, which is a challenging but laudable goal. Multidisciplinary refers to the idea that the study makes novel contributions to multiple disciplines. Take our example of the antidepressant fMRI study. If it is the study of a relatively novel drug with still unexplored mechanisms of action in the brain, it will be of interest to pharmacology. If it links two strong changes in thought and emotion, it may be of interest to psychologists and clinicians. If it involves novel innovations in data acquisition, it may be of interest in the field of MR physics. And if it involves novel computational methods to analyze brain networks, it might be of interest to the fields of computer science, informatics, and related disciplines. Not only is this difficult to pull off but also most studies should probably not try to be novel in so many different ways. However, the potential for innovation in multiple disciplines is one of the things that draw scientists from different areas together to contribute their expertise, creativity, and ideas.
Chapter 4 - Brain mapping: A conceptual overview
What is a brain map?
Understanding the basics of brain mapping is increasingly important for a broad segment of society as brain images make their way into media, medical practices, courtrooms, advertisements, and other sectors of public life. However, without an explanation of the process and some of the ground rules, our understanding of how we construct brain images and what they can and cannot tell us about the brain and the mind is not obvious.
Both functional and structural imaging rely on construction of brain maps, which are maps of localized signals. There are many types of brain signals that we can map which relate to many external (outside the brain) conditions and outcomes. However, different types of brain maps rely on many of the same principles and underlying assumptions. We will devote this chapter to a conceptual overview of how researchers construct brain maps, what we can learn from them, and what some of those assumptions and limitations are.
The brain maps like those shown in Figure 4.1, generally speaking, are statistical constructions. In some cases, brain images display actual data values; this is typical in neuroradiology, in which experts `read’ an image and come up with an opinion or diagnosis. However, in most scientific areas, researchers want to make quantitative inferences, which means statistically comparing image data across conditions or individuals and then showing maps of the statistical results. We often call this practice statistical parametric mapping. Such maps show brain areas where researchers have deemed some effect of interest statistically significant.
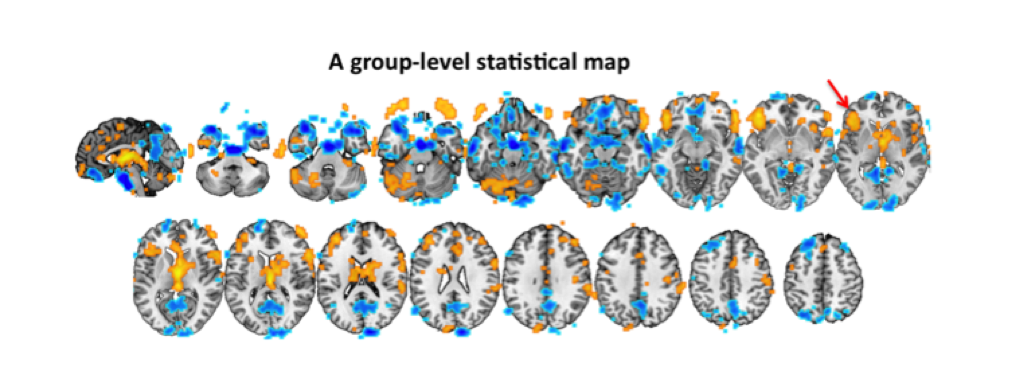
Types of maps
The types of processes that researchers map to local brain regions or networks are numerous. They include:
- Effects of experimental manipulations
- Correlations with behavior, clinical status, or other person-level outcomes
- Correlations with performance or other within-person variables
- Brain areas’ correlation with other specific areas
- Brain areas’ that are part of a group of areas (e.g. a cluster or network)
Accordingly, a first question to ask about any brain map is what effect it actually maps.
Types of inference
A second question to ask is to whom does the map apply - which individual or population of individuals? Data from only a single individual, scanned repeatedly, can be used to construct some maps as shown in Figure 4.2’s top panel. We refer to these as single-subject maps. These maps are common in some sub-fields, such as vision science or primate neuroimaging, and are increasingly present in clinical and legal applications. Researchers can construct single-subject maps by comparing data from one condition (e.g. one experimental task) with another across repeated measurements, which thus test statistical significance in each brain region or `voxel’ (a three-dimensional cube of brain). Another method to construct these maps is by comparing an individual with a population of other individuals. If the statistics are valid (a big if!), such maps can say something useful about how an individual’s brain differs from others’ brains.
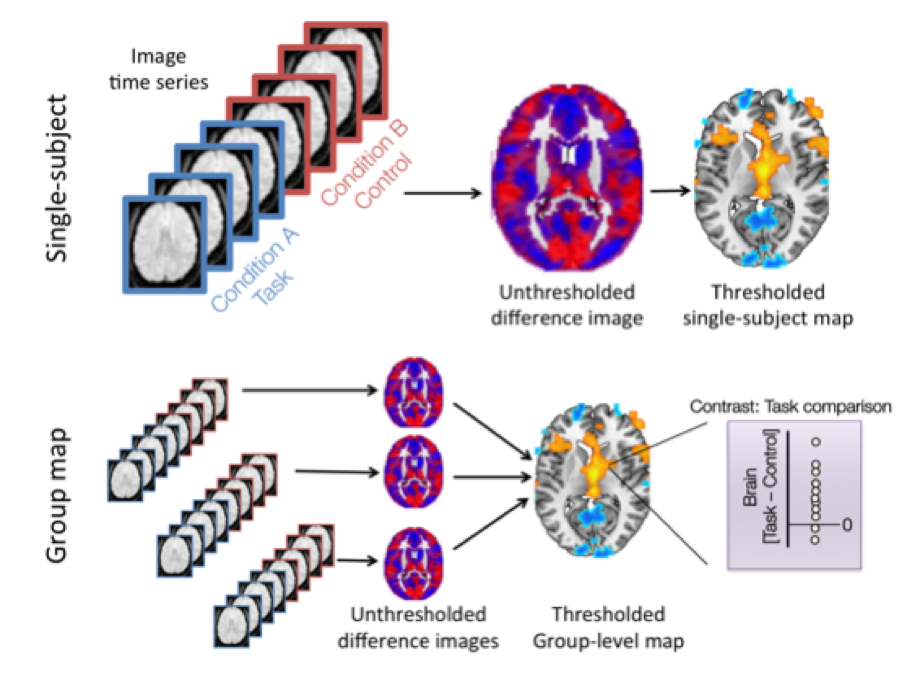
However, single-subject maps cannot tell us much about the brain’s general organization: researchers cannot use them to make population inferences, which are claims about how the brain functions in general. To make such claims, it is necessary to scan a group of participants and conduct statistical tests which explicitly evaluate how well the findings likely will generalize to new individuals. We refer to these as group-level maps; they identify brain areas that show consistent effects across individuals. The bottom panel of Figure 4.2 shows a schematic view of a group-level map’s construction. Technically speaking, such maps require a statistical procedure which we often call random effects analysis because the statistical model treats each participant as a random effect. We will return to this in more detail in later chapters. The map shown in Figure 1 is a group-level brain activity map.
Maps can widely vary in what they reflect, but they all share the same underlying basic distinction between single-subject and population inference. For example, Figure 4.3 shows maps of three different kinds of brain connectivity. In this case, the colored regions do not show the significant effects of interest; here the lines connecting regions show the effects: they indicate significant functional associations across regions. The left map comes from a dynamic causal model (DCM), which analyzes dynamic regional changes from one second to the next, while controlling for other regions and experimental task variables, to examine relationships among regions. Lines show significant associations at a population level. The center map comes from a method which identifies the most likely connections among regions and their variations across time. The connections the lines identify are not necessarily individually significant. This is common practice with many multivariate map types: one must be careful to make the correct inference because regions associated with a `network’ are not necessarily all significantly associated. Finally, the map at the right shows a large scale network in which each colored circle represents a brain region or system and each line shows significant associations across studies. Clearly, knowledge of a map’s construction process and its level of analysis are crucial for understanding what it means.
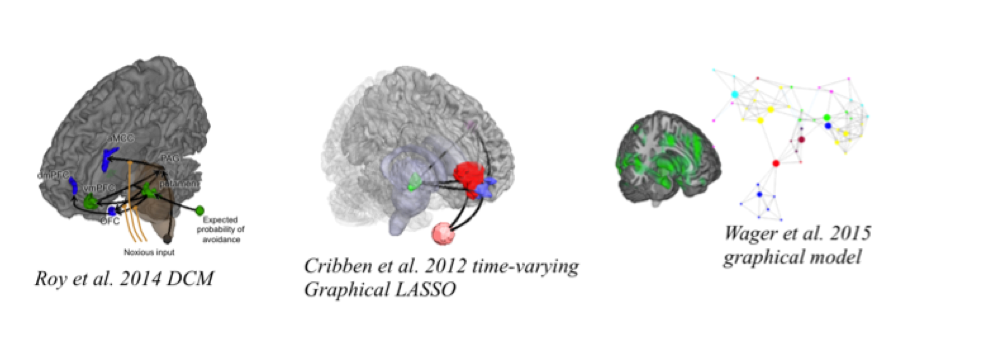
Fundamental assumptions and principles
In order to make statistical maps of all kinds, we rely on the assumption that the brain signals we measure reflect both effects of interest and noise. Researchers further assume that the noise is independent from the effects of interest (e.g. ``random’’). Repeated measurements in which the noise varies independently and stochastically allow us to obtain an average map that contains the true effect and reduces noise to a minimum. As the noise randomly varies around the true effect, it `averages out’, so the more data we collect, the closer the average noise will get to zero - as long as the noise is independent of the interest effect.
Consider the example in Figure 4.4. The brain - we show one representative horizontal slice here - contains some areas with a true effect, shown in blue. Perhaps this is a working memory task that requires people to maintain more versus less information in their minds; the map reflects concentration of the blue areas in frontal and parietal cortico-striatal networks. We observe a mixture of the true effects (signal) plus random noise, in red here.
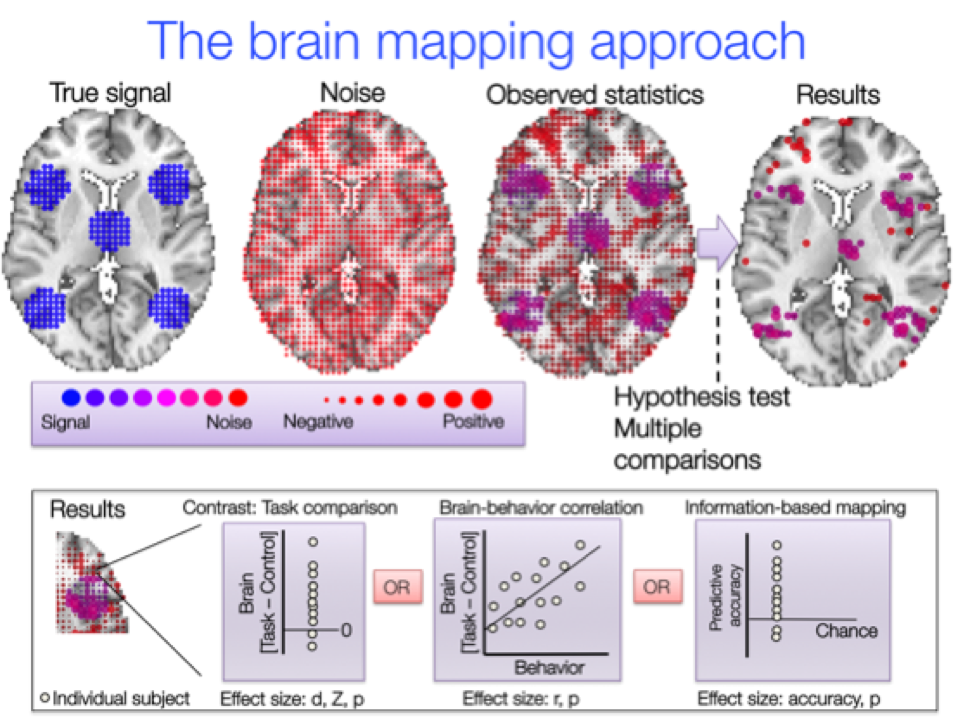
Importantly, this noise is non-zero even averaged across the observed data, so we need to first separate it from the signal and then decide which areas really show the effect. We do this with a statistical test which compares each voxel’s observed effect with its noise level (i.e. signal/noise). Common statistics, which include T-scores, F-values, and Z-scores, are all examples of such signal-to-noise ratios. We then compare the resulting statistic value with an assumed distribution to obtain each voxel’s p-value. The p-value reflects the probability of observing a statistic value (e.g. a T-score) as or more extreme then that actually observed under the null hypothesis - that is, if there is no true effect. The lower the P-value is, the less likely that we believe the null hypothesis is true. We compare p-values with a fixed value to threshold the map and to infer which voxels show true effects. Because of the many possible tests, researchers often set a very high bar for significance (i.e. low p-values) by correcting for multiple comparisons.
When we use standard statistic values like T-scores and compare them with their canonical, assumed distributions, we are using parametric statistics. When we use the data itself to estimate the null hypothesis’ Ñ which often involves fewer assumptions Ñ we are using nonparametric statistics.
In most cases, we test each voxel in the brain separately, ignoring other voxels’ potential influence, to construct brain maps. This is the case whether one maps activations which respond to a task, structural differences between groups, or functional correlation of areas with a `seed’ region of interest. It is a big assumption that the rest of the brain doesn’t matter, so many multivariate analyses relax this assumption in certain ways (depending on the specifics of the multivariate model). However, the assumption is in some ways quite useful as we can interpret one brain area’s effects independently of other area’s responses. For example, a brain map which correlates activity levels in an anger-induction task with self-reported anger levels can provide a simple picture of which areas are associated with anger and so can be a starting point for more sophisticated models.
This basic brain mapping procedure applies to the vast majority of published neuroimaging findings, including both structural and functional imaging using MRI and PET. Figure 4.4’s bottom panels show three common data types that go into such maps. On the left, the statistical brain map’s voxels reflect a task-related group analysis that compares a task of interest to a control task. Each data point that goes into the test at that voxel (the circles) is the [task - control] contrast magnitude from one participant; the null hypothesis here is that the population’s [task - control] differences are zero. The center map shows a brain-behavior correlation in which the test statistic is the correlation between the activity levels (often in a [task - control] contrast) and an external outcome, as in the anger example above. The right map shows an ``information-based mapping’’ test in which the test statistic is the average accuracy in predicting a stimulus category or behavior from each voxel’s local multivariate patterns of brain activity. In all of these cases, the above principles and assumptions apply.
Bringing prior information to bear: Anatomical hypotheses
Regardless of the type of map constructed and the variables involved, researchersÕ basic question is, ``is there some effect at this location?’’ As Figure 4.5 shows, researchers can apply hypothesis tests to each brain voxel or to a set of voxels in pre-defined regions of interest (ROIs). They can also apply hypothesis tests to voxels in a single ROI or to signals averaged over voxels in one or more ROIs. These examples illustrate a progression from conducting many tests across the brain to performing few tests, a movement that depends on the prior information brought to bear to constrain hypotheses.
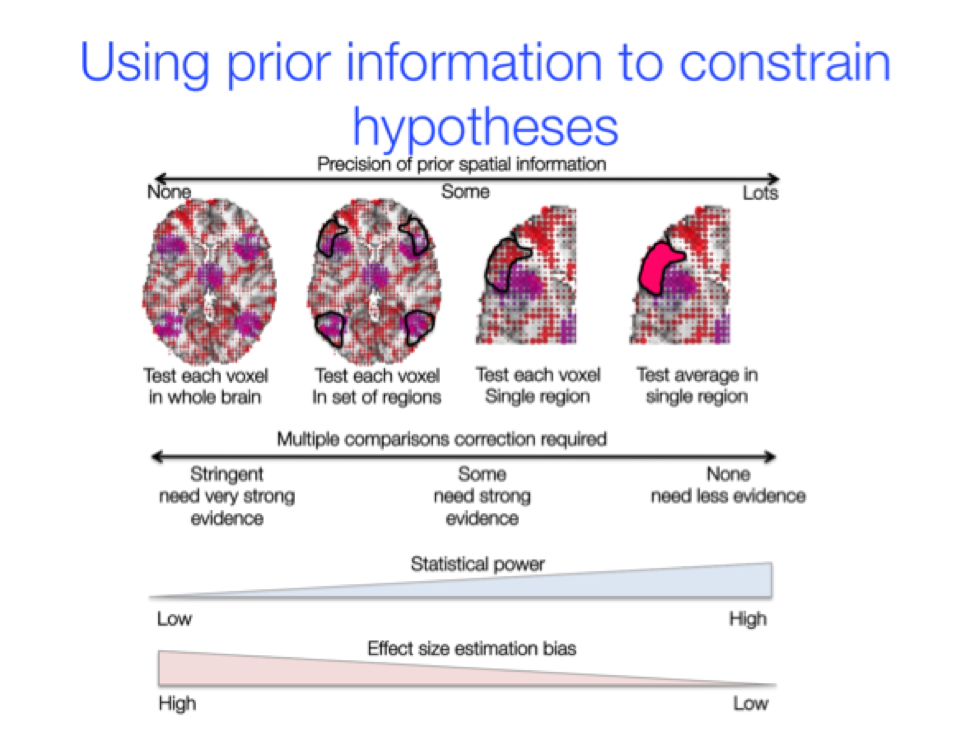
The more tests researchers perform then the more stringent the correction for multiple comparisons must be if they are to interpret all significant results as `real’ findings. As the threshold becomes more stringent, statistical power - the chance of finding a true effect if it exists - drops, often dramatically, which entails increasingly missed activations. In the extreme case in which there is only one ROI and the signal in its voxels is averaged, researchers perform only one test and do not need multiple comparisons correction.
Researchers need not limit a priori hypotheses to single regions; it is also possible to specify a pattern of interest, in which an average or a weighted average is taken across a set of brain region, and a single test is performed. Figure 4.6 shows an example from a working memory study. We first defined a pattern of interest based on previous working memory studies from neurosynth.org, which is an online repository of over 10,000 studies’ activation results. Then we applied the pattern to working memory-related maps from two participant groups - a group exposed to a social evaluative threat (SET) stressor and a control group - by calculating a weighted voxel activity average in the pattern of interest. Applying the pattern allowed us to (a) establish that, in our study, working memory produced robust activation in the pattern expected from previous studies and (b) test for SET effects on working memory-related activation without needing multiple comparison correction.
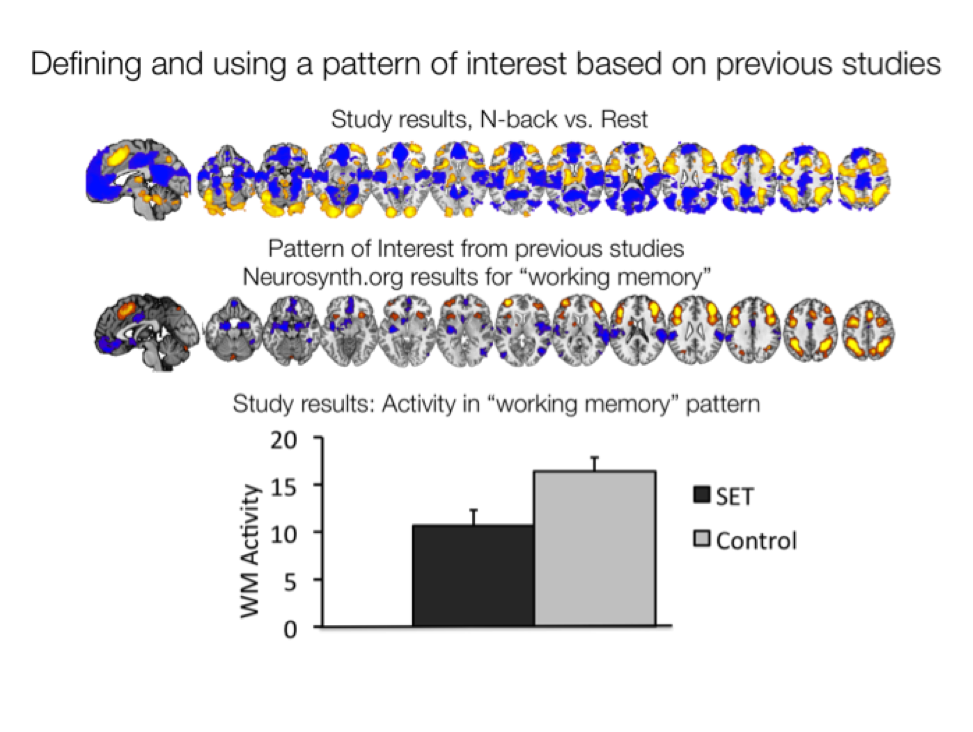
There are thus many benefits to specifying anatomical hypotheses a priori. However, when we specify a priori hypotheses, we must truly specify the region or pattern in advance based on data whose errors are independent from the dataset testing the effect, otherwise the p-values and the inferences will not be valid. We suspect there are many unreported cases of post-hoc ``a priori’’ selection of ROIs.
Types of inference: What brain maps can and cannot tell us
What can we infer from thresholded brain maps of all types, regardless whether they concern anatomy, neurochemistry, or functional activation? What we can make inferences about is rather specific and may not be exactly what you expect. Below, we discuss inferences about brain effects, a term which applies to many types of images that span beyond task-based activation, anatomical relationships with behavior, or maps of molecular imaging. First we discuss inferences about brain effects’ presence, size, and location. Then we discuss forward and reverse inference, which, respectively, relate to making inferences about the brain and our psychological states (or other outcomes).
Inferences about the presence, size, and location of effects
The basic brain mapping procedure involves a test of significance at each voxel; this is a hypothesis test. This allows us to reject the null hypothesis that a subset of voxels has no effect in favor of an alternative hypothesis. That alternative hypothesis, however, is not very precise: it is merely that there is some non-zero effect.
As we will see in the next chapter, this does not let us conclude anything about how big or how meaningful the effects are; attempts to do so using standard hypothesis testing procedures can be highly misleading. At best, then, brain maps can allow inference that a set of significant voxels has some effect, but not how much effect.
Standard brain maps are also not very good for determining which voxels do versus do not show effects. Thus they are not useful to show us the complete pattern of activity (or structural effects, etc.) across the brain. This is primarily because of the stringent thresholds that usually limit the false positive findings. Current thresholding procedures do not optimally balance the number of false positives and false negatives (missed findings).
Another thing in which standard brain maps are not particularly good for is precise determination where the effects are in the brain. This may seem very surprising as researchers nearly always interpret thresholded brain maps in terms of where the most statistically significant results lie in the brain. However, the trouble lies in brain maps providing confidence intervals (which researchers use as a guide for how strongly to believe in the effect) on whether each voxel is significant but not on the significant voxels’ locations. They provide a `yes/no’ value for whether a significant effect appears at each voxel. Inferences about result locations, then, are heuristic rather than quantitative.
This limitation becomes intuitive if we consider the brain map in Figure 4.1. The map contains significant activation (yellow) in the ventrolateral prefrontal cortex (vlPFC), marked with a red arrow. Imagine repeating this experiment again. What are the chances that the exact same voxels in vlPFC would be active? Or that the most active voxel would fall in the exact same location? We do not know. Standard mapping procedures do not provide p-values or confidence intervals on the activationÕs location or shape. However, we know from meta-analyses like the one in Figure 4.7 that the location of the peak voxel will likely be quite variable, possibly around plus or minus 1 Ð 1.5 cm. Incidentally, Figure 4.7 does show spatial 95% confidence intervals for the across study mean location for positive (green) and negative (red) emotions, drawn as 3-D ellipsoids. In addition to noise related uncertainty about local effects’ locations and shapes, we also must keep in mind that artifacts and imprecision in anatomical alignment can also cause mis-localized effects. All brain images have an intrinsic point-spread function, or a blurring of localized true effects at one local brain point into a broader `blob’ of observable signal. BOLD images in particular are susceptible to arterial inflow and draining vein artifacts; they are also typically overlaid on an anatomical reference image which may not perfectly align with the functional map.
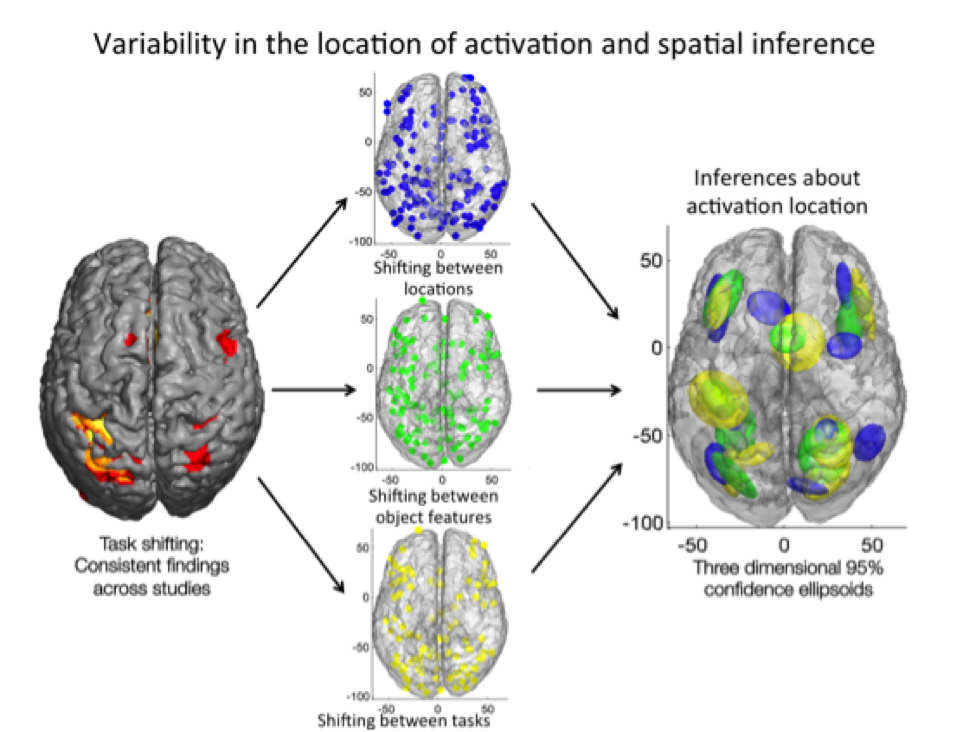
The upshot of all this is that though we can make inferences about certain areas’ activity, we must be cautious about over-interpreting size and location of significant findings and about the completeness of the picture thresholded maps provide.
If these types of inferences sound limited, we agree! Standard brain maps are very limited - we devote much of the next chapter to further unpacking their limitations. Fortunately emerging alternative methods avoid some of standard brain maps’ problems. These include (a) specific multivariate pattern analyses types that build predictive models and (b) spatial models that we can use to make inferences about the location of effects.
Forward and reverse inference
Inferences drawn from brain maps have another limit. Typically, researchers either (a) induce a psychological state by manipulating experimental variables or (b) observe a behavior of interest or other outcome. Then researchers assume that the state or behavior is known and make inferences about the statistical reliability of brain activity given (or conditional on) the state or behavior. In Bayesian terms, we infer the probability of brain activity given a psychological state or behavior, or P(Brain | Psy). This is forward inference, which can tell us about how the brain functions under different psychological or behavioral conditions but not much about the psychological state or behavior itself (see Figure 4.8).
Standard brain maps provide information on forward inferences. Though above we expressed them in terms of probability, the same concept applies to effect size measurements. The stronger a brain map’s statistical effects then the more likely we are to observe a significant result in probabilistic terms.
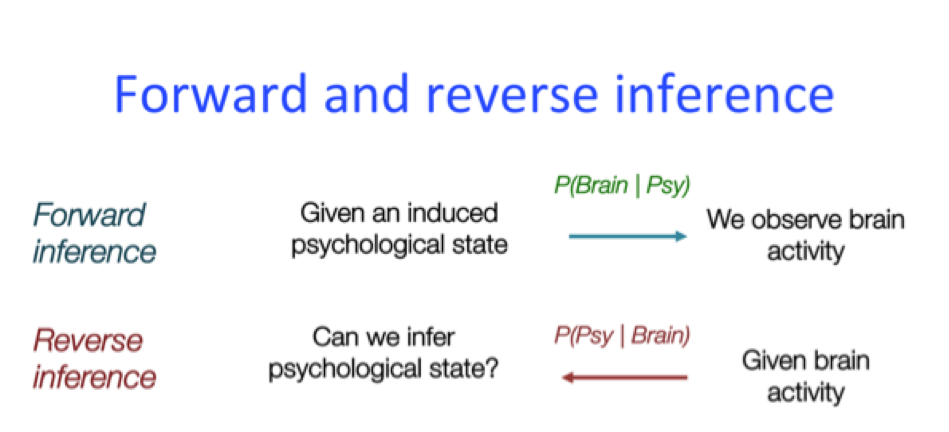
Why can’t standard brain maps teach us much about psychological states? Forward inferences take psychological states as given. They do not tell us how brain measures constrain our theories of which psychological processes are engaged. For that, the inference we want concerns P(Psy | Brain), the probability (or, heuristically, the strength) of a psychological process’ engagement given activity in a particular brain region or pattern. Neuroimaging literature has termed this reverse inference. Though related through Bayes’ Rule, forward and reverse inference are not the same thing qualitatively or quantitatively.
The field of logic calls fallacious reverse inference ‘affirming the consequent’. For example, assume this statement is true: ‘If one is a dog, then one loves ice cream’, or P(Ice Cream | Dog) = 1 for short. Then given that Mary loves ice cream, i.e. P(Ice cream) = 1, one might erroneously infer that Mary is a dog. The problem is that all dogs love ice cream, but not all ice-cream lovers are dogs. P(Ice Cream | Dog) = 1 does not imply that P(Dog | Ice cream) = 1.
Standard brain maps’ limitations in constraining psychological theory have led many researchers to be critical of neuroimaging, often rightly so. Examples of papers that make fallacious reverse inferences - like, for example, inferences that long-term memory processes were engaged (Psy) because the hippocampus was activated (Brain) - litter neuroimaging literature. In fact, some psychologists have argued that neuroimaging has not taught us anything about the mindÑyet.
Reverse inference is actually possible; it is a major piece of the puzzle in constraining psychological (and behavioral and clinical) theory with brain measures. To understand how, let’s revisit forward and reverse inference from a diagnostic testing perspective. P(Brain | Psy) is the ‘hit rate’ of significant activity given a psychological state; testing theory calls it sensitivity. In a standard test, e.g. a diagnostic test for a disease, Brain is analogous to having a positive diagnostic test, and Psy to having the disease. P(Psy | Brain) is the test’s positive predictive value - how likely one is to have the disease given a positive test. High positive predictive value requires both high sensitivity and high specificity, which entails a low probability of a positive test if one does not have the disease - or, in brain imaging terms, low P(Brain | ~Psy), where ~ means `not’. To use a brain example, before we can infer that hippocampal activity implies memory involvement, we must first show that hippocampal activity is specific to memory and that other processes do not activate it.
Thus to make reverse inferences about psychological states we must estimate the relative probabilities of a defined psychological hypotheses set given the data, typically by using Bayes Rule. This requires analysts to construct brain maps of multiple - ideally many - psychological conditions and assess the brain findings’ positive predictive value formally.
In addition to assessing positive predictive value, analysts can optimize maps and models of brain function to maximize function - that is, to strongly and specifically respond to particular classes of psychological events, behaviors, or other prompts. This is the goal of an increasing number of studies which use multivariate pattern analysis with machine learning or statistical learning algorithms. This is a promising direction; we devote a great deal of space to these techniques later in the book.
Ability to infer a psychological process’ presence or strength is important in its own right. It opens up various possibilities for testing and constraining psychological theories - or at least, their biological bases. Valid reverse inferences could allow, in some cases, researchers to infer a number of processes otherwise problematic or impossible to confidently measure. Among others, these states include being in pain, experiencing an emotion, lying or hiding information, and engaging in cognitive work. Researchers can use reverse inferences to probe the unconscious and to help study mental processes in cognitively impaired, very young, or otherwise unresponsive individuals. And, finally, comparing brain markers for different psychological processes could allow us to develop new mental process typologies - including emotion, memory, and other processes - which, regardless whether they match our heuristic psychological categories, may have their own diagnostic value.
In conclusion, standard brain maps provide specific types of inference about brain activity. Though there are a number of fundamental limits to these inferences, new techniques are circumventing many of those limitations and providing a more complete range of inferences about the brain and mind.
In the next chapter, we further explore those limitations, some ways that researchers exploit brain maps to support erroneous conclusions, and how you can become a savvy consumer of neuroimaging results.
Chapter 6 - How to lie with brain imaging
In this chapter, we explore the dark side of neuroimaging results. We discuss several fallacious arguments for which to watch out. In writing this, we are inspired by two classic books. One is called ‘How to lie with statistics’, which, of course, really tells you how you should not lie with statistics or at least how to avoid being fooled by those who do. The other book is Bob Cialdini’s terrific ‘Influence’, in which he claims that his own gullibility inspired him to study persuasive power and resistance. Accordingly, this is not really a chapter about how you can lie with brain imaging, in case you were wondering. It’s really a chapter about what not to believe.
Below, we describe five tricks to make your results look specific, strong, and compelling, and also to make them come out like your theory predicted. For example, if you have a theory that requires two psychological tasks to produce highly overlapping brain activity, we can help you make that happen. Or if your theory specifies that patients and controls engage very different brain systems, we can help with that too.
Of course, these are not the only ways to lie with brain images. There are the obvious ways - plain old making stuff up or engaging in a little self-deception like defining ‘a priori’ ROIs after peeking at the statistical maps (because you would have expected activation in the precuneus, right?). There are also techniques like ‘P-Hacking’, which include sleights of hand such as continuing data collection, adding and removing covariates, or transforming outcome measures until you have a significant result. We’ll discuss those more later. Here, we’re interested in techniques that are, at least in some cases, a little bit more subtle and that apply even to brain maps generated through otherwise valid means.
How to tell a story about the ``one brain region’’
The high-threshold
Most clinical disorders and many processes which psychology studies are likely distributed across multiple brain systems. How can we make such a bold claim? To be encapsulated in one brain region, a process must be relatively pure, which implies that localized lesions produce complete and specific deficits. This is true in a few cases: V1 lesions produce cortical blindness and specific inferior temporal lesions produce prosopagnosia, a face recognition deficit. But most processes, even evolutionarily conserved and sensory-driven ones like pain, are highly distributed. The trouble with this is that a neuropsychological tradition which focused on selected cases of specific deficits after focal lesions created a past ‘culture of modularity’. Prestigious journals like Nature and Science have historically vastly preferred simple results with one-point headliner messages like ‘this brain region implements this complex psychological process’ (we won’t pick on any specifics). So how do you get your results to tell that simple story?
The answer is very simple: the high-threshold. Simply raise the bar for statistical significance until you have one region (or very few) left in your map. Not only is this useful for writing a paper around a single brain region which enables emotion, goal setting, attention shifting, hypothesis testing, or whatever you’re studying but it is also really useful if you see significant activation in the white matter or the ventricles - places you shouldn’t see activation in artifact-free statistical maps. The antidote is to (a) choose the threshold a priori and (b) require researchers to show the entire map, including the ventricles (or at least to check it).
How to make your results look really strong
Strong results mean large effect sizes which include high correlations between healthy-sized, meaty-looking blobs with bright colors and brain measures and outcomes. There are two techniques to ensure your brain map looks as it should no matter how weak the effects actually are.
Circular selection (this technique is also known as the voodoo correlation)
Let’s face it: most complex personality traits and clinical symptoms are unlikely to strongly correlate with any one brain voxel. The reliability of both brain and outcome measures limit such correlations’ true values. The heterogeneity of outcome measures also limits them: there is no single reason why people feel depressed, experience neuropathic pain, or are schizophrenic, courageous, or optimistic. Additional limits include person-level factors which affect brain response magnitude unconnected to outcomes of interest: among these factors are individual differences in hemodynamic responses and vascular compliance, blood iron levels, alertness, and caffeine intake. However, isn’t it more convincing if your brain findings correlate with optimism or anxiety above ?
Yes, virtually any study can achieve this. The procedure is simple: first run a correlation map across the whole brain, then select the peak region and test that region’s correlation. If your sample contains 16 participants, then any voxel with a p-value less than 0.005 will show a correlation of at least or so. Now, maybe you’re worried about not finding any voxels with such a low P-value…. but don’t be. If you test only 1,000 independent comparisons, you have a 99% chance to get at least one significant result, even with no true signal anywhere in the brain.
Add to this that brain maps can easily contain 100,000 voxels, though they are not independent. And, of course, if you have some voxels with more modest true correlations - say, in the
range - then the chances are even greater that you will select a voxel with an apparent
correlation, or higher. Small sample sizes will increase your success, too, because they are more variable across the brain. With only 8 participants, the average significant voxel at
will correlate above
.
There is more good news as well: this technique will work for any effect size measure whether it is a correlation, a difference between experimental conditions, or a multivariate pattern analysis classification accuracy.
If you do not want others’ circular selection to fool you, you will need to know that (a) there was a priori selection of all tested regions and (b) the report includes all tested effects. And keep in mind that (c) if there are many tests, some will show large effects by chance.
The low-threshold extent correction
Circular selection will make your effects look really strong, but won’t create those large, fruit-colored blobs on your brain map. Such blobs are important because human minds naturally confuse ‘lots of reported areas’ with ‘strong effects’, even if the two are unrelated. The solution is to lower the statistical threshold until you get large blobs - and possibly to mask out the pesky white matter and ventricle activations that tend to appear at low thresholds. The problem is that reviewers are savvy and will ask you to report results with multiple comparisons correction.
There is a method to lower your statistical threshold and still claim rigorous multiple comparisons correction. How is this possible? Fortunately the technique called cluster extent-based correction lets you set as liberal a `primary threshold’ as you want (say, uncorrected) and then correct for multiple comparisons based on the extent of the blob . Among other problems, correction methods are too liberal with such low thresholds (http://www.ncbi.nlm.nih.gov/pubmed/24412399). The bonus is that your figures’ maps will show all the voxels significant at the liberal, uncorrected threshold even though you can at best actually only claim that the activated area has some true signal somewhere in the activated area.
This antidote to this trick is to use more stringent primary thresholds, to clearly indicate each significant region’s identity in figures, and to make it evident that most voxels which appear in the figure may not actually be activated. Or, of course, to avoid extent-based thresholds altogether.
Overlapping processes: How to make two maps look the same
The overlap zoom-in
Let’s say that your theory focuses on overlap across two or more processes such as two types of emotion, pain, or cognitive control. You scan two tasks and compare each one’s activation maps with its respective control condition. To support your theory, simply focus on the overlapping voxels and assume non-overlapping ones are due to noise. Now even if the maps are 95% different across the brain, you can still claim support for your theory. You might also do a multivariate `searchlight’ analysis that looks explicitly for similar brain regions across the two processes. Anything significant in the map is positive evidence and the remaining brain areas in which the tasks are dissimilar are just inconclusive null results attributable to low power.
If you are not getting enough overlap, the low-threshold extent correction can greatly amplify the extent of your activation patterns and thus increase the apparent overlap. Hopefully most reviewers will not realize that this is not a valid test as your comparison is between two maps with `some true signal somewhere’ at each individual voxel, as though each voxel were significant. And, finally, to enhance any of these techniques, you can make a figure that focuses selectively on the overlap locations.
The antidote of this technique is to provide unbiased similarity measures across the whole brain including regions that might be shared or unique. Such approaches are not common in neuroimaging literature yet, which makes this technique particularly hard to counteract.
The low-level control
If the overlap zoom-in does not provide enough ‘evidence’ for overlapping activation, try this additional technique. Similarity is relative: an apple and a banana are dissimilar when compared to an orange but are quite similar when compared to roast beef. Likewise the technique for making the activity maps of two tasks very similar is to compare them to a very dissimilar control condition. Of course, reviewers might object if you compare your two tasks to a third which is very dissimilar. Fortunately, however, there is a perfect comparison condition that will not raise eyebrows, namely rest.
Imagine you have a theory that altruism is an automatic human response (which it actually may be). You posit that punishing others produces internal decision conflict even if they deserve it. Thus you would like to demonstrate that brain responses are similar when unfairly punishing others and within a cognitive ‘conflict’ task. No problem. Simply compare each to rest then look at the overlap of the resulting activation maps. Many low-level processes will activate in each map: processes involved in most cognitive tasks such as orienting attention, making basic motor decisions, and executing them. If your study is sufficiently powered, you will observe beautiful overlapping activation in areas including the anterior cingulate, the anterior insula, and the supplementary motor cortices.
The antidote to this technique is to require tight control of the tasks or, even better, to track parametric strength increases of each process in which you are trying to assess the overlap. Then the maps you compare will be more tightly constrained to reflect the cognitive processes of interest.
How to make two maps look really different
Now let’s assume that you have the opposite problem. Your theory dictates that different processes should be involved in two or more maps. Perhaps you suppose that children with attention deficit disorder process cognitive stimuli differently than those without the disorder. No matter how similar the underlying brain processes are, you can always conclude that the activation patterns are distinct if you so desire.
The high-threshold can come to your aid again here. Because every brain map is variable and the locations of significant voxels vary, the chances that any two maps will produce overlapping voxels decreases as the threshold increases. Alternatively the low-threshold extent correction can also be helpful as it will produce large blobs in mostly non-significant constituent voxels. If you focus on the differences in maps rather than similarities and zoom in on areas with apparent differences, then you will be able to convince most readers that the activation maps are quite distinct. If you analyze the spatial patterns, e.g. by correlating the maps across voxels, then the noisier your maps are, the more likely your maps will be uncorrelated.
The antidotes here involve spatial tests, analyses in which one generates a p-value for whether two tasks activate distinct brain locations, and treating participants as a random effect (we discuss this in more detail later). Without spatial tests, no principled null hypothesis exists for how many voxels should or should not overlap in two truly similar underlying processes, so you can essentially say whatever you want. However, if a reviewer should require you to do a spatial test, you would need to demonstrate statistically significant differences in the activated areas’ location or shape. This is a much higher bar to pass, especially considering that interpreting the voxel overlap heuristically is really no bar at all.
There are also antidotes which relate to spatial pattern tests. Reviewers may require you to demonstrate that each of the two patterns you correlate (a) are reliable, with high correlations with themselves or related within-task measures at re-test and (b) strongly correlate with a task state or outcome. If so, then the bar is again raised: a null correlation across tasks is contextually meaningful with positive correlations within-tasks.
Conclusions
With this chapter, we hope we have been able to show you that you can take valid, albeit noisy, statistical brain maps and shape their presentation to fit your theories in multiple ways, independently of the truth. Of course, we do not want you to actually do this (in case anyone missed that point). We want you to be aware of these deceptions and self-deceptions to ensure that your analyses are unbiased so data shapes theory rather than the opposite. The best way to make sure this happens is to care more about discovering something true than about finding supporting evidence for a particular view or theory. This is sometimes difficult when the truth does not line up with our publication goals and cherished beliefs, but it is the austere path of science.
Chapter 7 - fMRI basics: Processing stages, terminology, and data structure
fMRI basics
In this chapter, we’ll talk about analysis of functional magnetic resonance imaging, or fMRI, data. We’ll start with some nomenclature, talk about data types and structures, and end with a little bit about fMRI data analysis goals. fMRI is a noninvasive technique for studying brain activity. By noninvasive, we mean that it generates pictures of the inside of your head without using any implants or injections. There are also no known side effects of being scanned frequently with fMRI. For example, one of our colleagues scanned himself nearly 100 times with no apparent adverse effects. Scans are now also routinely performed on both infants and children.
A single session in the scanner allows researchers to collect many image types, both anatomical (or `structural’ MRI) and functional (related to dynamic brain activity changes). Here, we are concerned particularly with functional imaging.
During the course of an fMRI experiment a series of brain images are acquired, often while the subject performs a task set. Those ‘tasks’ can include cognitive paradigms, viewing, hearing, feeling, tasting, or smelling various stimuli. An increasingly popular ‘task’ is simply lying in the scanner doing nothing; there is now a whole subfield devoted to ‘resting-state’ fMRI.
Processing and analysis stages
Figure 7.1 shows a basic flowchart for a typical fMRI experiment. Throughout this book, we’re going to keep coming back to this flowchart to unpack each of the basic data processing and analysis steps in more detail. All studies begin with experimental design, which is perhaps the single most crucial factor in determining how well the experiment will go. There are a number of design principles rooted in statistics which apply to all design types, neuroimaging or otherwise. Other principles are specific to fMRI experiments and relate to the properties of fMRI data and their specific analyses.
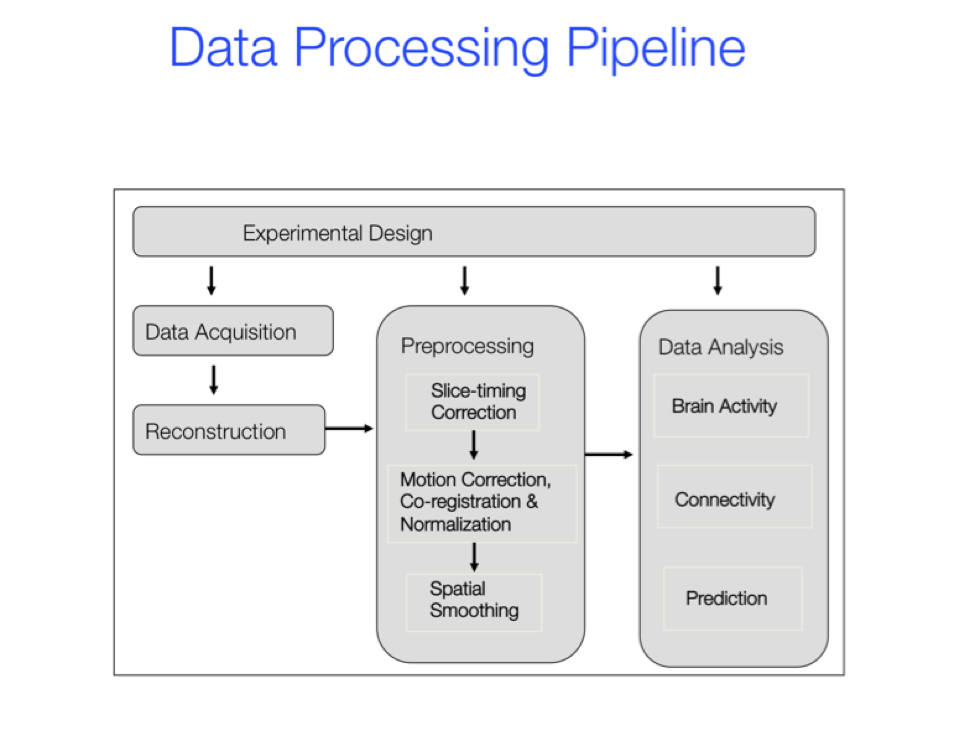
Data acquisition is the next step after experimental design, followed by reconstruction of the data into images. Researchers perform a series of preprocessing steps before statistical analysis. These deal with anatomical alignment of the various image types (coregistration), timing issues (slice-timing correction), head movement (motion correction), and image transformation onto a standard anatomical reference space (spatial normalization or warping). Researchers also commonly perform artifact-mitigation procedures and physiological noise correction. After preprocessing, the images are ready for statistical analysis. Analyses can test task- or outcome-related brain activity, assess functional connectivity, and develop multivariate predictive models designed to correlate optimally with experimental variables or outcomes.
Acquisition
We acquire MRI and fMRI data by applying radiofrequency (RF) pulses to the brain. These pulses perturb the magnetic spins of the protons of hydrogen atoms (mostly in water molecules) so they give off energy with particular spatiotemporal characteristics. The RF antenna reads off this signal, which is then used to reconstruct images. During data acquisition, magnetic gradient coils are applied in particular patterns so signals from different spatial locations in the image are given particular characteristics, which enables accurate spatial reconstruction. The pulse sequence, the software that runs the RF antenna and gradient coils which acquire the signal, determines what type of data the process acquires - including whether the image is structural or functional. This will all be covered in greater detail in a later chapter.
It’s useful to know some basic terminology related to MR image acquisition. Figure 7.2 shows some of the basics. The bounding box that defines the image acquisition volume depends on the field of view (the slice dimensions), the number of slices, and the slice thickness. Data are sampled within small cubic volumes called `voxels’ or volumetric pixels. Voxel size depends on slice thickness and in-plane matrix size, which is the number of grid elements on which each slice’s data are sampled. The field of view divided by the matrix size is the in-plane resolution, measured in mm. Thus in-plane resolution and slice thickness determine voxel size. Researchers typically desire isotropic voxels, which are the same dimension on all sides, though unequal sizes work as well. A typical size is voxels; this is close to optimal for many purposes when using a 3-Tesla scanner.
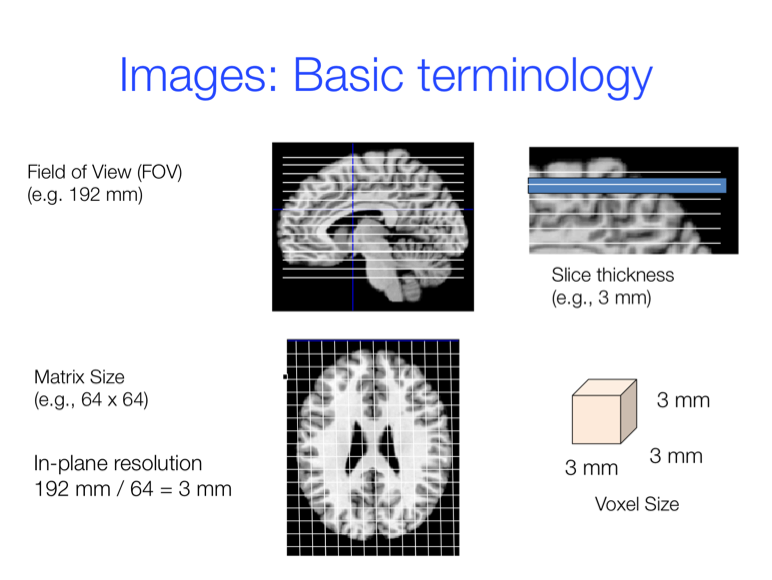
Designing an fMRI study requires a series of tradeoffs given the study’s particular goals. One fundamental tradeoff is between spatial and temporal resolution. You can either collect data with high spatial resolution or collect data fast, but you can’t do both. Spatial resolution defines our ability to distinguish how an image changes across different spatial locations and thus also our ability to extract location-coded information about brain states and behaviors. Both the voxel size and the image’s underlying smoothness (blurriness), which depends on the main magnetic field’s strength and gradients and on underlying physiological limitations (because most of our signal is blood flow-related), determine spatial resolution. Temporal resolution determines our ability to separate brain events in time. Both the TR and the hemodynamic response to neural and/or glial events’ time course (more on this is below) determine this.
Image orientation and dimensions
Understanding and interpreting which part of the brain one is viewing requires some practice. The brain is a complex three-dimensional structure with many curved Ã’CÓ shape sub-structures that wrap around the brain’s center, the thalamus. It is typical to show neuroimaging results on anatomical brain slices. Figure 7.3 provides a basic orientation to those slices and their spatial relation to the overall head and brain surface. Each of the three dimensions of brain space has a special name. The left-to-right dimension is conventionally the direction in standard brain coordinate space. The back-to-front dimension is the
dimension which ranges from posterior at the brain’s back to anterior at the front. Sometimes anterior is also called rostral, which means ‘toward the head’, and posterior is called caudal, ‘toward the tail’. The bottom-to-top dimension is the
dimension which ranges from inferior to superior locations. These locations are sometimes also called ventral (‘towards the belly’) and dorsal (‘towards the back’).
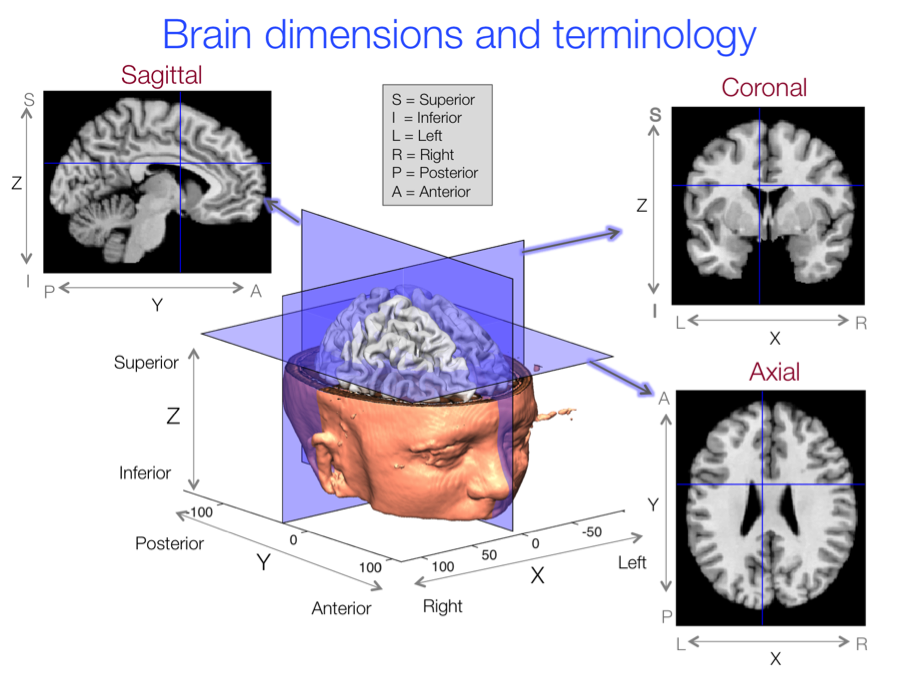
Researchers typically report locations along these dimensions in coordinate triplets with
,
, and
values indicating distances in millimeter units relative to a zero point. The
point is, by convention, the anterior commissure, a small white-matter bundle which connects the brain’s two hemispheres. Figure 7.4 shows this point.
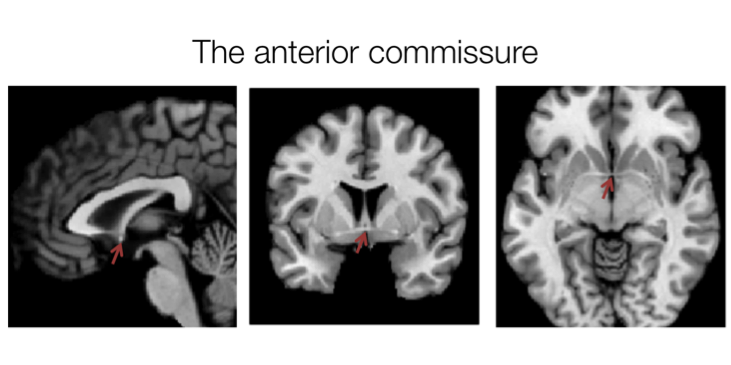
In the brainstem, some of the dimension names are not very intuitive because they describe the dimensions as in an animal which walks on four legs with the spinal cord toward the rear (caudal) and the midbrain, which lies just below the thalamus, at the rostral end. Thus, the part of the brainstem toward the back of the head is the dorsal brainstem and the part toward the front is the ventral brainstem. Figure 7.5 shows these directions and some of the most important structures’ locations.
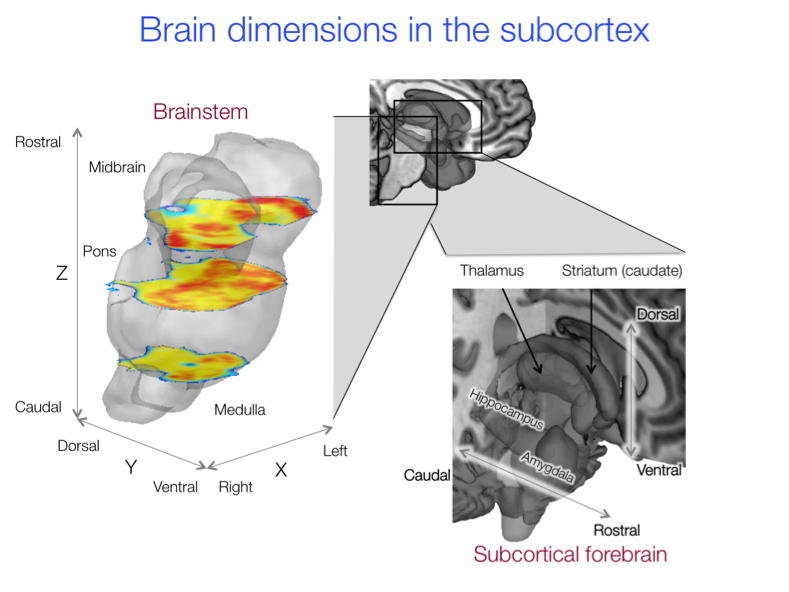
The sections of the brain that are typically used to display neuroimaging results have particular names too. Figure 7.3 shows these. Coronal slices are sections that span the left-right and inferior-superior dimensions at one location from front to back. Sagittal slices span the front-to-back and inferior-superior dimensions at one location from left to right. And axial or *horizontal * slices span the left-right and front-to-back dimensions at one location from inferior to superior.
fMRI time series
Functional images (also called -weighted images) have lower spatial resolution than structural images. That is, they’re much blurrier than their structural counterparts. However, we can measure many of them, so they have higher temporal resolution and we can use them to relate signal changes to experimental manipulations or other outcomes that vary from second to second.
One participant’s fMRI dataset contains a time series of 3-D images, or `volumes’, shown in Figure 7.6. The volumes often cover the entire brain but can also cover just one brain tissue section or slab at a higher spatial resolution. The data for each volume are usually acquired slice-by-slice; after completing one volume, the scanner moves on to the next image. As they are collected, the data are sampled onto a rigid voxel grid.
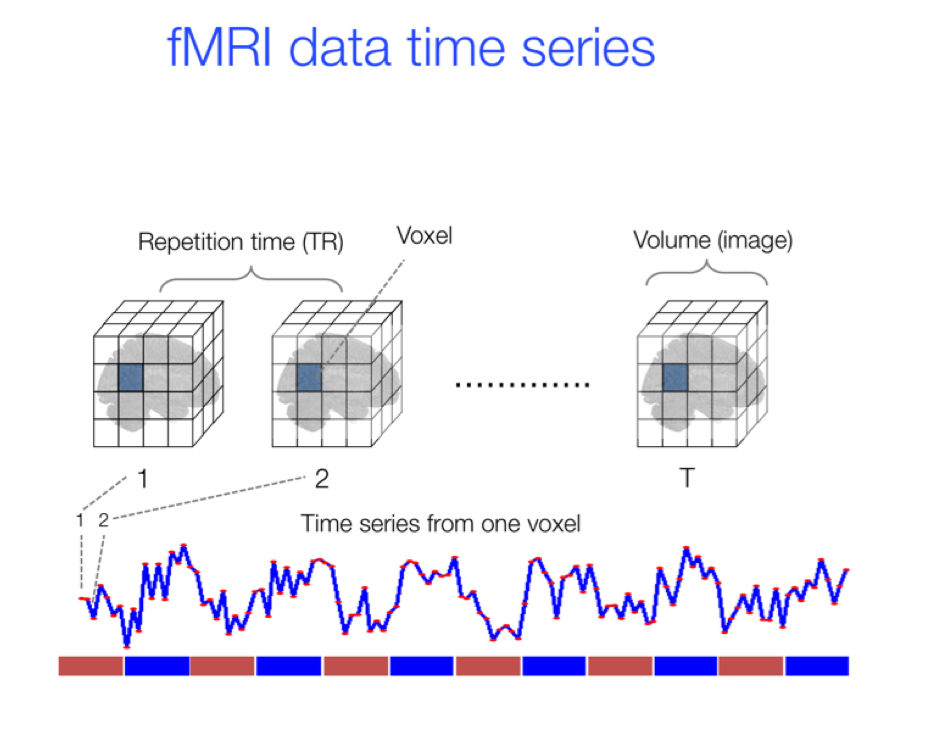
It is not uncommon for each volume to contain 100,000 or more voxels, though the number varies depending on the acquisition choices. The pulse sequence, or the software that runs the radiofrequency antenna and magnetic coils which acquire the signal, determines how researchers acquire data. The repetition time between volumes, or TR, varies quite a bit across studies but typical values for a whole-brain acquisition have historically been about 2-3 seconds. However, recent imaging advances now make it possible to collect a whole brain volume in . Experiments can be brief at 6 minutes of functional time (e.g. 180 volumes), but experiments that include 40 or more minutes of functional time, with over 1,000 volumes measured at a typical TR, are not uncommon in practice.
Thus fMRI data comprise hundreds to thousands of images in a time series. As local regions’ oxygen metabolism and blood flow change, researchers use fluctuations in the measured signal to make inferences about brain activity and connectivity. The usual approach towards assessing brain activity is based on examining average fluctuations locked to particular experimental conditions or events. We refer to this as task-based fMRI. Researchers assess brain connectivity by examining associations in the fluctuations among voxels with or without task condition influence analyses.
A simple, canonical example of a task-based fMRI experiment is a motor task. LetÕs say we want to examine activity increases in the motor cortex when participants execute simple finger movements. Researchers often use such tasks as quality control assessments in order to check signal and analysis quality. Participants might alternate between 20-second long blocks of finger tapping and 20 seconds of rest. This is a `block design’, illustrated in Figure 6.6’s bottom panel. Not all designs are equally efficient or powerful, but 20-second long blocks have good properties in particular; we will return to this concept in later chapters.
Statistical analysis
Once we have run an experiment and say obtained motor task data for a group of participants, we are ready to analyze the data. Recall that the fMRI dataset contains a time series of each voxel’s signal values. A basic analysis will study each participant’s data one person and one voxel at a time. fMRI data are quite noisy, so we use statistical analysis to determine whether a signal change is consistently associated with the finger-tapping task.
The first step of statistical analysis is to fit a model to each voxel’s time series. In this case, the model simply states that activity levels are different between finger-tapping and control periods. We can use a t-test to examine how large the difference (or `contrast’) is between finger tapping and rest divided by the noise measure (i.e. error variability). Then we make a map of the resulting voxel t-values and their associated p-values, which provides evidence to evaluate the null hypothesis of no task effect. Again, each voxel corresponds to a spatial location and has an associated statistic that represents the evidential strength for task-related effects. Researchers usually threshold these maps by applying a statistical cutoff related to the p-value, so scientific papers only plot and discuss voxels with sufficient evidence of an effect.
The description above covers the basics of a simple statistical analysis. However, it leaves out one key detail. fMRI activity, either BOLD or ASL, does not rise instantaneously when the task begins. Rather it increases over several seconds as blood flow function increases, peaks at about 5-6 seconds after the increase in local brain metabolic demand and decreases after 10-15 seconds. This function over time is the hemodynamic response function or the HRF which researchers must measure, or else assume a canonical function, to be able to perform a reasonably accurate analysis. Figure 7.7 shows a canonical HRF widely used as a model for fMRI responses. One piece of good news is that even very brief neural events (e.g. a stimulus presentation) can reliably elicit measurable hemodyamic responses, so fMRI can be sensitive to short events. Another is that even with a complex neural event series or sustained blocks as in our finger-tapping experiment, we can still account for the HRF in our analysis.
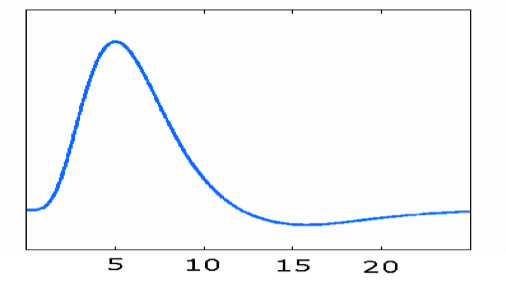
The description above highlights the fact that fMRI data analysis is fundamentally a time series problem. However, it’s a time series problem on steroids because every voxel has its own time series and there are about 100,000 voxels. The concept of analyzing voxels individually is sometimes called mass univariate analysis; in this, we treat all of the voxels separately then construct a map of the statistical results at each voxel. Other techniques that do not separate the voxels are becoming more widely used. We typically refer to these techniques as multivariate analyses because they are multivariate in brain space and model multiple voxels simultaneously.
Clearly fMRI data analysis is a massive data problem. Each brain volume consists of roughly 100,000 different voxel measurements. Each experiment might contain 1,000 brain volumes or more. And we might repeat each experiment for multiple subjects, maybe 20, 30, or 40, but sometimes hundreds or thousands, to facilitate population inference, i.e. making generalizable conclusions about human brain function. Because of both the amount of data and its complexity, fMRI data statistical analysis is challenging. The signal of interest is relatively weak and the data exhibits a complicated temporal and spatial noise structure. Thus there are ample opportunities to develop new increasingly sophisticated and powerful statistical techniques.
Data structure in fMRI experiments
Hierarchical data structure
fMRI data has a hierarchical structure, as Figure 7.8 shows. Understanding this structure and dealing with it appropriately is important when undertaking fMRI data preprocessing and statistical analysis.
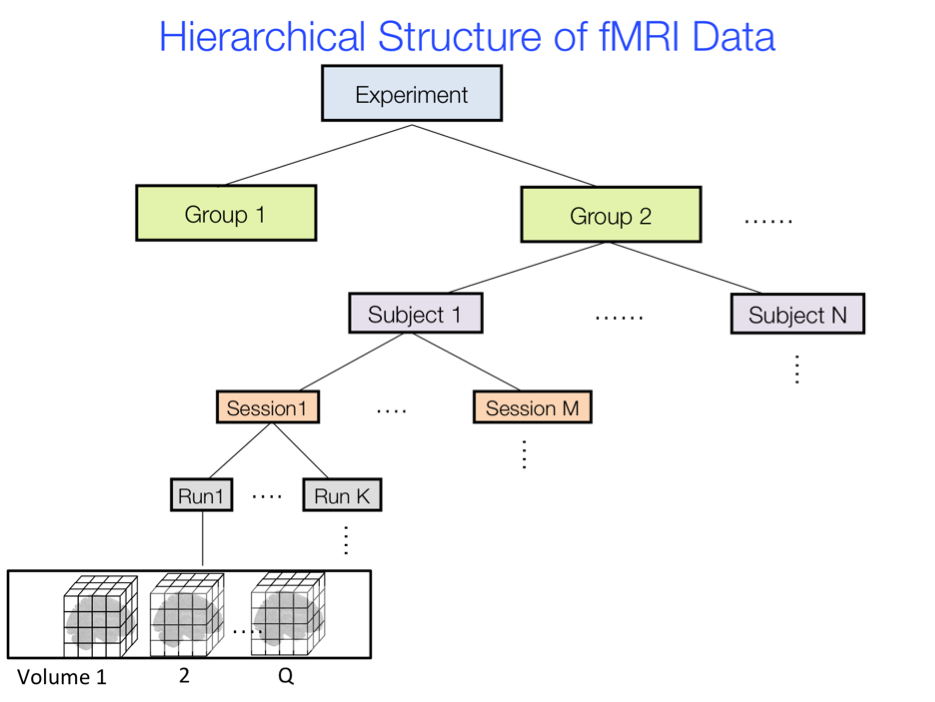
The vast majority of experiments include many different participants’ data. This is critical in order to obtain population generalizable results - i.e. results that are not just idiosyncratic features of the individuals we happened to study but rather that constitute general conclusions that apply to new individuals. Each participant (sometimes called `subject’) performs the same task or tasks. Sometimes we nest participants within groups, such as patient group versus controls or elderly individuals versus young. In other cases, there is just one group and researchers’ interests are in studying experimental manipulations, behaviors, or other outcomes measured within-person. Even if we do not organize participants into groups, it is still possible relate brain activity differences to individual person-level variables (e.g. age, performance, or other variables).
Experiments entail collecting many repeated measurements on each participant over time. We may scan each participant longitudinally in multiple sessions. During a session, it is typical to start and stop the scanner multiple times, collecting data for brief periods - usually 4-10 minutes. We refer to these as runs. Because head movement during the scans is particularly problematic, short runs are advisable to give participants a break and allow them to communicate with the experimenters if necessary (though speaking can induce additional head movement!). Each run, in turn, entails a series of brain volumes, one per TR, nested within task conditions (e.g. finger tapping and rest). Each volume consists of multiple slices acquired sequentially, and each slice contains many voxels.
Often we analyze each participant separately with a mass univariate analysis of each voxel’s experimental effects. This is a first level analysis. The resulting maps of experimental effect magnitudes, called contrast maps, become the data for a second level analysis of effect reliability across participants, which includes differences between groups and effects of individual differences.
Image file formats
One barrier to entry in fMRI analysis is that the image data are not simple text files. Rather they are stored in specific customized formats along with associated `meta-data’ or information about the imaging parameters.
Historically, data formats differed widely across scanner manufacturers and software packages. For example, raw data on General Electric scanners are in a proprietary format called `P-files’ and on Siemens scanners as DICOM files (which stands for Digital Imaging and Communications in Medicine, http://www.dicomlibrary.com/dicom/).
DICOM files contain a single slice’s data at a single time point, with extensive `header’ information, though different scanners use and store this differently. A study can thus include millions of files, which presents logistical challenges with many file management systems. Therefore preprocessing and statistical analysis packages usually require that we convert these images into other, standard formats.
Though these standard formats also differed across packages, most now have the facility to read and write NIfTI (which stands for Neuroimaging Informatics Technology Initiative) images, a standard 3-D or 4-D file format. A 3-D NIfTi file contains one image per volume, while a 4-D NIfTi file often contains a single person’s time series of image volumes. Both these files have a .nii extension. A related older file format less standardly used across software packages is the Analyze image format which has .img extensions and associated separate header files containing meta-data with .hdr extensions.
It is important to exercise caution when reading and writing files across various software packages because these packages use the meta-data differently. Some researchers thus feel uncomfortable about mixing and matching algorithms from different software packages, though it can be done if one is cautious and meticulous.
One of the biggest issues of which to be aware relates to flipping images in the direction, from left to right. The brain is largely symmetrical, which makes it difficult to tell if an image display’s left side, such as in Figure 7.9, is on the left or right side of the brain. The fact that there are two orientations typically used to view images further complicates this point of potential confusion. If the image display is in radiological format, the brain’s left side is on the displayed image’s right side. This display is as though one looks up at a person’s brain from their feet. If images are in neurological format, the brain’s right side is on the displayed image’s right side. This is the format most cognitive neuroscience research uses. Though imaging software should keep track of format, different packages use header information related to flipping differently and custom reconstruction and stacking code at different research centers can also treat the image orientation information differently. As a result many, many errors have undoubtedly occurred.
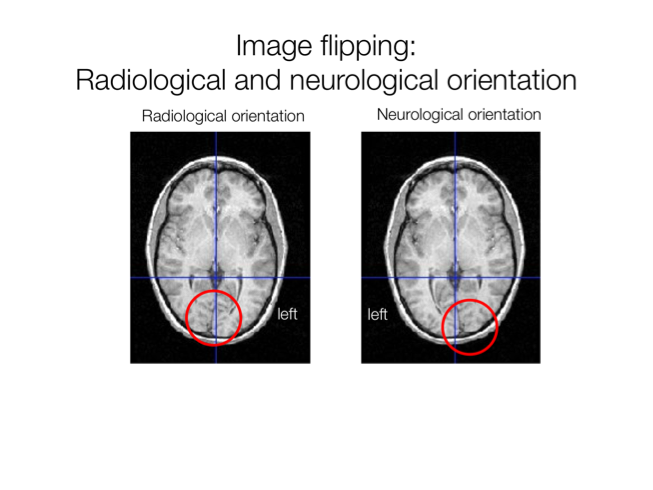
A number of strategies can help avoid this error. Using NIfTi format helps, as does consistent use of a single software package. Researchers often tape a Vitamin E capsule to the same side of each participant’s head. This produces a bright spot on the image in one hemisphere. Finally, we can heuristically check the flipping by viewing the images, because the left occipital lobe’s larger size in most people causes the calcarine fissure’s deviation to the right as it courses from front to back. This asymmetry is prominent in the structural image shown in Figure 6.9.
Conclusions
In this chapter, we briefly covered the major steps in fMRI data processing and analysis and some of the most commonly used terminology. In addition, we reviewed the hierarchical structure of fMRI data and common image file formats. In later chapters, we will cover the design, acquisition, preprocessing, and statistical analysis of fMRI data in more detail.